Why is Context Relevant in Big Data ?
- Jeffrey Wallk
- Oct 13, 2013
- 2 min read
Updated: Apr 11, 2019
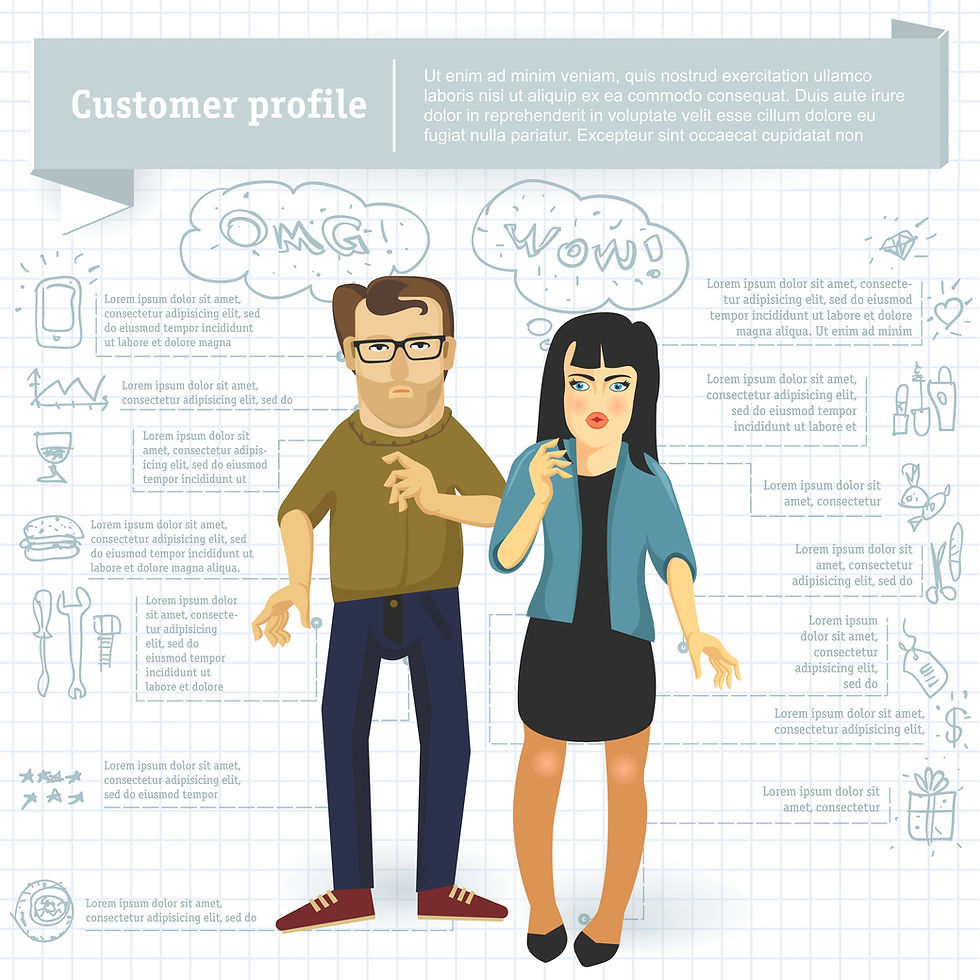
A recent discussion was started in an online group that I frequent. The original post asked the question about the importance of contextualizing Big Data. There may be a few more "dimensions" to the question, which are worth considering.
One covers the point that data is not just a discrete resource. It's actually a living asset, which has a lifecycle which spans from acquisition (through one or more sources) to the cleansing and warehousing of the data and eventually to one or more transformations where it may be merged and shaped into metrics, reports, and analytics. And, perhaps one day the data may be deemed useless and eventually terminated.
Another point is that it can be helpful to distinguish between Master Data and Metadata, though Master Data is typically viewed as a subset of Metadata. Mastered Data is focused primarily on the attributes used to contextualize transactional data, which must be accurate to support enterprise resource planning (ERP) engines, used used by financial and operational management and reporting across the organization. The superset of Metadata is much larger and is not as well structured (or managed typically), so separate sets of tools are used to perform pattern based discovery.
Modeling and managing the context and semantic perspectives within complete scope of Metadata is both a challenge and opportunity for information architecture. Contextual relevance provides a vast set of patterns that support stakeholder points of view (POV), where each POV makes the data meaningful for individual consumption. The same lifecycle approach applies to this data, but we are still in the early stages of discovery tools and the ability to continuously improve this type of data. The growing importance of AI (machine learning, neural nets, and deep learning) are creating more urgency for organizations to ensure these additional dimensions of data, semantics, and alignment with perspective are accurate and relevant (suggesting temporal and contextual attribution).
The current practices and tools used to data management and data integrity will need to be extended and/or augmented with new technologies since there are far too many dimensions and complexities with this expansive (and fast growing) set of metadata for traditional manual governance. Automated governing tools an analytics will need to be developed to assess the data accuracy for the metadata, which will likely involved quality driven statistical analysis to provide on demand data assessments to be incorporated into the probability analysis and algorithms supporting AI.
Eventually AI will be required to assess itself and all of the inputs that it relies upon as a part of a recursive quality and learning platform. As this emerging capability evolves, it may create new opportunities for organizations and open doors for sharing / brokering of Metadata (which may prove more valuable than transactional data in the near future).
Comments